How do recommendation engines for fashion eCommerce work?
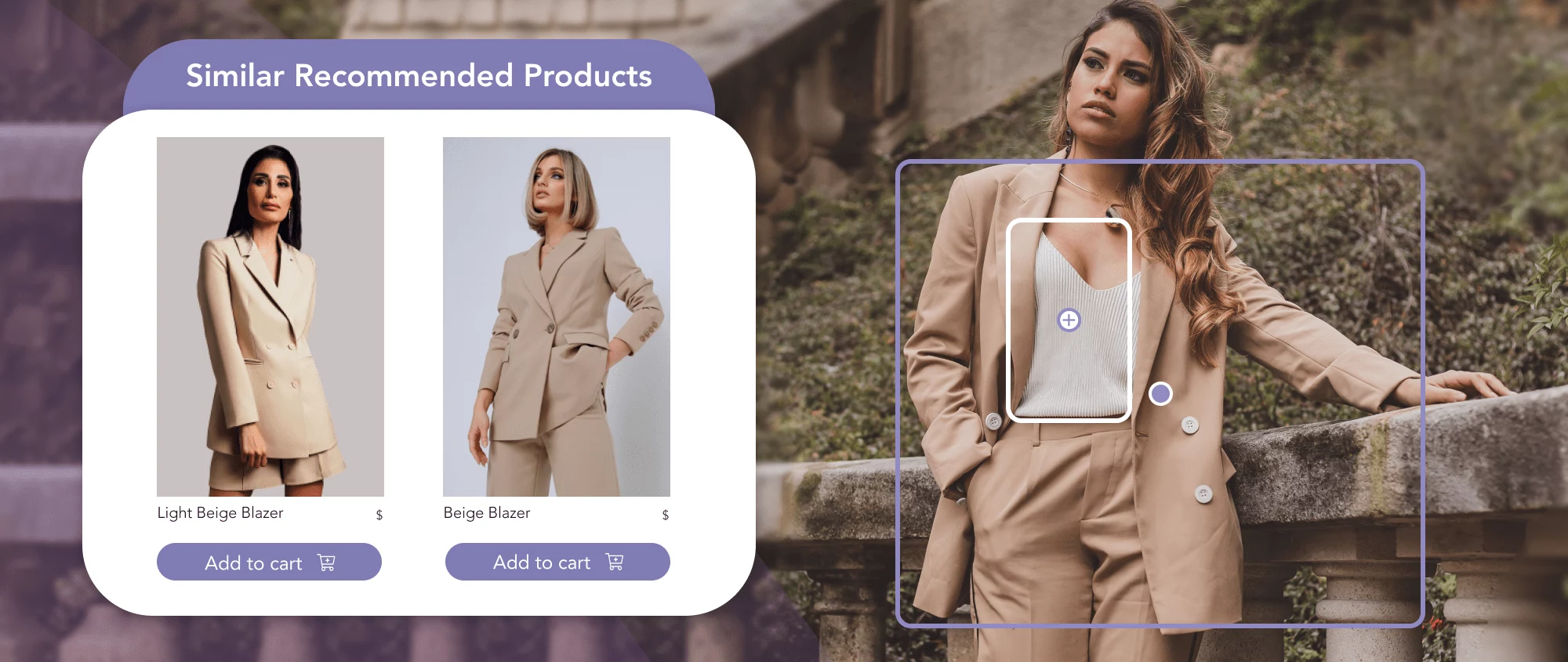
Imagine having a shopping buddy who always understands your shoppers’ preferences and offers just the right items—without being overbearing. That virtual assistant is a recommendation engine, a tool that can be integrated into your website in minutes to enhance your customers' shopping experience. But first, let’s explore how these engines work and how they benefit fashion eCommerce businesses like yours.
What are product recommendation engines?
Product ecommerce recommendation engines are sophisticated filtering systems that predict and display items a shopper is likely to buy. These systems analyse user behavior, purchase history, and product similarities to suggest relevant items, making ecommerce recommendations more personalised and effective. Instead of leaving customers to search aimlessly, they proactively bring products to the forefront, tailored to individual preferences.
Today, ecommerce recommendation engines are a must-have for any eCommerce business, especially in fashion, where personal tastes matter deeply. They enable personalised shopping experiences, increasing engagement and boosting average order value through effective fashion product recommendations. A great recommendation engine ensures your customers feel seen and understood—key ingredients to a fantastic shopping experience and essential components of effective e-commerce growth strategies.
It’s no accident that retailers like Zara and ASOS achieve high sales—AI-powered recommendation engines play a crucial role. 😉
How do recommendation engines in eCommerce work?
Product recommendations in eCommerce are powered by machine learning and AI algorithms. Thanks to the automated configuration and management, the shopping recommendation engine can intelligently choose which products to offer to a specific customer. Different systems work in different ways, but each product recommendation engine follows the same logic that can be simply explained in four phases.
1. Data collection
The engine collects all the relevant and available data. It can collect data in an explicit way by collecting ratings and comments on products or in an implicit way by looking at search history and previous interactions.
2. Data Storing
In this phase, the recommendation engine stores the collected data for each customer. The engines usually use large SQL servers to store the information.
3. Analysing matches
By cross combining the collected data and the products in the eCommerce store, the engine comes up with similar matches.
4. Filtering
In the last phase, the engine filters the findings and finally offers the most relevant products to each potential customer.
Types of recommendation engines
Based on the filtering, ecommerce recommendation engines can be classified into three main types.
- Collaborative filtering
- Content-based filtering
- Hybrid recommendations systems
Let’s deep dive into each of them.
Collaborative filtering
Collaborative filtering happens when a system analyses customer behavior and predicts what they might like based on similarities with other customers. This recommendation type assumes that people who showed interest in something in the past will eventually show interest in similar items again.
For example, let’s say that two girls are shopping in Pull and Bear. Mary bought a red hoodie, blue jeans, and black sneakers. And Anna bought blue jeans, black sneakers, and a brown backpack. The collaborative filtering engine will assume that they have similar tastes and styles, and Anna would also like the red hoodie and Mary would like the brown backpack. So, they’ll see those products in the recommendations section on the online shopping site.
Content-based filtering
This type of filtering is based on the product’s description and the profile of the customer’s preferences and choices. In this system, the product’s description contains keywords to support the description, including fashion product attribution, and the user’s profile holds the items that the customer likes.
The recommendation engine collects the customer’s preferences and makes a profile. Then the algorithm tries to recommend other products that are similar to the already liked ones.
Imagine that you’re reading an article about fashion for Grammy’s 2022. The engine will process that information and will recommend similar articles, for example, an article about fashion for the Academy Awards and another one about copying Zendaya’s look.
The logic behind this is that if a customer likes a particular item, they will probably like other similar items.
Hybrid recommendations system
As the name suggests, this recommendation system uses a combination of both collaborative and content-based filtering to find the perfect products that the customer will like.
This type of recommendation in eCommerce works by collecting and comparing the searching habits of similar users as well as offering products that share characteristics with the products the customer liked and rated lately.
Let’s clear it up.
Imagine this situation: Toby and Dave are shopping for Nike running shoes. Toby looked for Pegasus 38 and Revolution 6. Dave liked Pegasus 38, Revolution 6, and Flex. The recommendation engine will show Toby Flex (collaborative filtering element) Pegasus 39 and Revolution 5 (content-based filtering element).
This type of filtering and product recommendation offers the widest spectrum and brings the shopping experience and personalisation to another level, as it is closely tied to a well-organised fashion product taxonomy, ensuring that recommendations are accurate and relevant.
Advanced Product Recommendation Tactics for Fashion eCommerce
In addition to common recommendation techniques, here are advanced strategies that can further optimise your fashion eCommerce business:
Leveraging User-Generated Content (UGC)
UGC is a powerful tool for fashion brands, as 65% of consumers purchase based on it. By incorporating customer photos or reviews directly in your product recommendations, you create social proof and authenticity. A branded hashtag campaign can encourage users to share their looks, which can then be displayed as shoppable content on your store’s homepage or product pages.
Shopping Cart Recommendations
The shopping cart is a prime opportunity to suggest complementary products that increase Average Order Value (AOV). By suggesting items frequently bought together or offering promotions such as “Spend X more for free shipping,” you create additional purchase incentives. This technique not only enhances upsell opportunities but also promotes impulse buying.
Search as a Recommendation Tool
With 43% of shoppers using search bars on fashion websites, optimising search as a recommendation tool is vital. By suggesting popular or trending search terms as soon as the search bar is clicked, you guide customers towards relevant products. Furthermore, you can suggest products even on no-results search pages to retain customers and avoid lost sales.
Exploratory Recommendations for Fashion Shoppers
Not all shoppers know exactly what they want. Implementing exploratory recommendations for new arrivals, best-sellers, or trending items helps guide indecisive shoppers. Displaying these on the homepage or collection pages ensures a more personalised browsing experience, encouraging customers to explore your brand’s fashion-forward offerings.
Recommending Products in Marketing Communications
Post-purchase emails, abandoned cart messages, and promotional SMS notifications can all feature personalised product recommendations. By showcasing complementary or similar items to what the customer has viewed or purchased, you increase the likelihood of repeat purchases. Personalisation in marketing communications boosts engagement and drives conversions effectively.
The Role of AI in Fashion Recommendations and Trends
The future of fashion eCommerce lies in leveraging AI-powered ecommerce recommendation engines that not only suggest products but also anticipate fashion trends. Here’s how modern AI systems are shaping the industry:
- AI-Driven Trend Prediction
Recommendation engines don’t just rely on historical data anymore. They use real-time market insights, social media trends, and even fashion runway data to predict what styles and products will be in demand next season. - Hyper-Personalisation
AI is pushing the boundaries of personalisation. These engines now analyse micro-behaviors—such as hover time, scroll patterns, and even the colors a customer seems to prefer—allowing for recommendations that feel tailor-made for each shopper. - Visual Search and Image Recognition
Fashion recommendation engines are integrating visual search features, allowing customers to upload photos of outfits or accessories and instantly find similar products in your store. This AI-powered image recognition takes product discovery to new heights, especially for fashion-forward shoppers. - Sustainability and Ethical Recommendations
More consumers are leaning towards ethical and sustainable fashion. AI-powered recommendation engines are beginning to incorporate sustainability filters, suggesting eco-friendly and ethically produced products that match shoppers’ values.
How can recommendation engines improve product discovery?
.png)
Regardless of which product recommendation type you use on your eCommerce store, recommendations will significantly improve and boost product discovery. Think of it like a virtual assistant that stands next to your customers, understands their desires, and offers similar products that they maybe couldn’t notice themselves.
Here are some ways in which recommendations improve product discovery and the overall shopping experience. Leveraging AI product discovery tools can further refine these recommendations, making it even easier for shoppers to find what they want.
- Showcasing best-sellers and featured products
- Helping customers to understand what they really want
- Boosting product visibility by showing the products more often
- Getting a higher conversion rate
- Increasing the engagement and customer interaction with the product
Suggested read: Product Discovery: A Practical Guide for Ecommerce
Conclusion
A recommendation engine is here to help your customers what they really want and help them discover new products they will love. By using an ecommerce product recommendation engine to suggest relevant products and provide enhanced product content, you’re ensuring a seamless shopping experience that will convert users into returning customers.
Pixyle.ai’s similar recommendations will help you offer an even more personalised experience to your customers. It will allow you to improve product visibility, increase the conversion rate, and make highly relevant offers.